Solutions
Automated Data Quality Monitoring
Achieving high coverage of your data ecosystem with data quality checks is hard, and relying on teams of engineers to write every single data quality check from scratch is unscalable.
Qualytics generates data quality checks based on your historic data’s shapes and patterns, enabling you to easily deploy these checks to catch anomalies and providing over 90% coverage from day one.
Inferred DQ Checks
Profiling automatically generates data quality checks based on the metadata generated. Typically 3 to 5 checks are generated per field, with additional checks generated for cross-column and cross-datastore relationships. As your data evolves over time, automatically update checks to
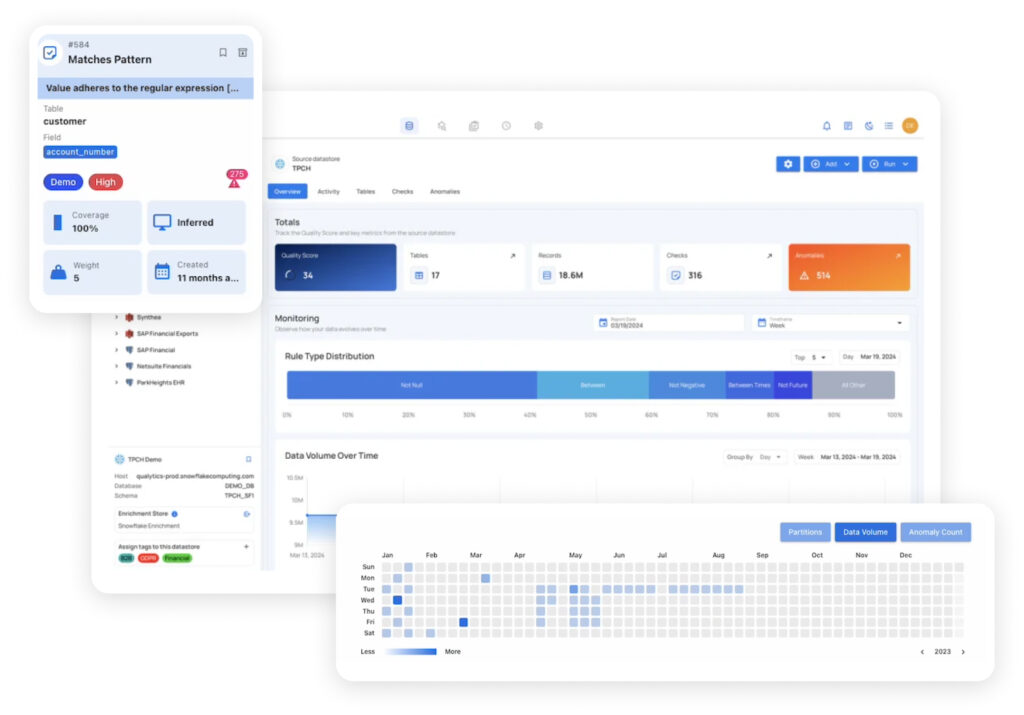
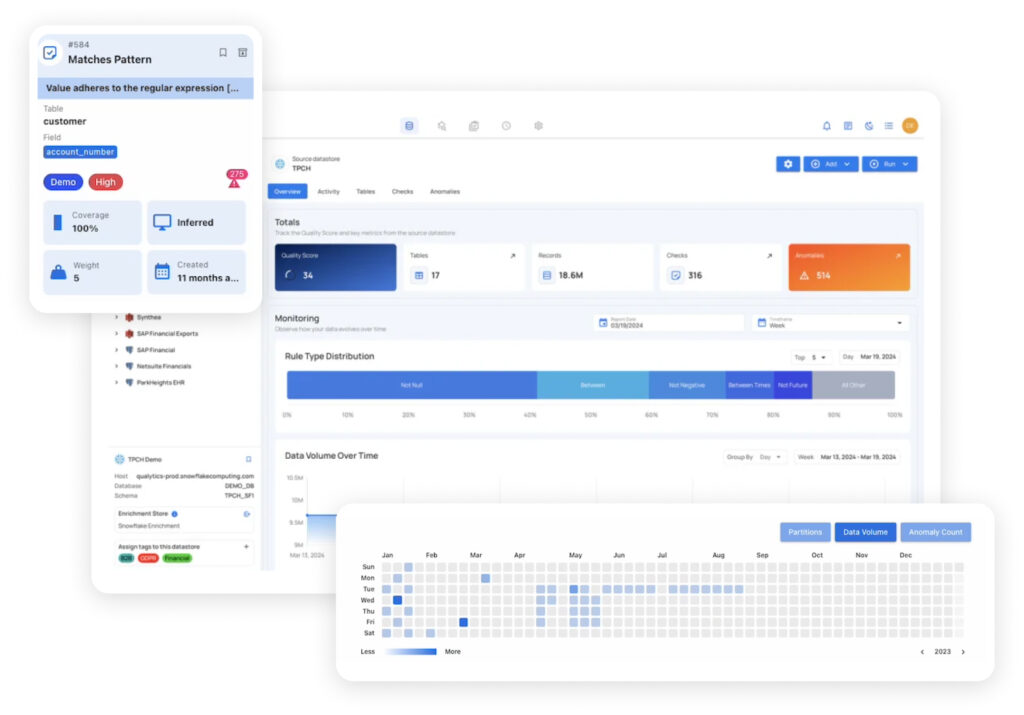
Give Feedback
Data Quality cannot only be reliant on machine-generated rules. Human input is important at crucial points to maintain and update checks with business context. We strongly believe that managing data quality at scale means giving supervised learning feedback to anomalies – and let the platform maintain check updates for you.
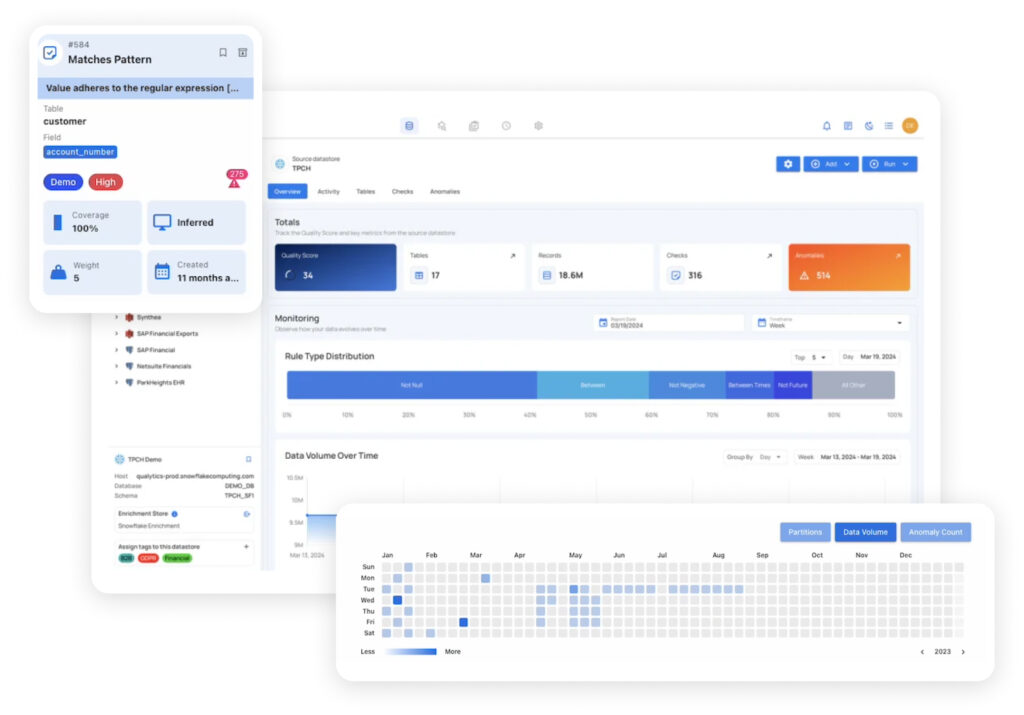
Generate Tighter Rules
Some data quality checks are reliant on relationships between multiple fields. For instance, for each market segment, the business context may change with the ranges of an acceptable value range. Qualytics enables users to define Group By pivots, enabling tighter rules to be generated.