Enterprise Data Quality Platform
Activate and operationalize your Data Quality at scale through advanced AI-driven rules and our intuitive low-code interface.
- 95% Automated Rule Generation
- Consistent, Simplified, and Seamless Governance
- Real-time anomaly detection across your entire data pipeline
- Track 8 Data Quality Dimensions
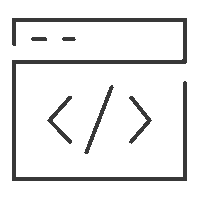
Automated Technical & Business Rules
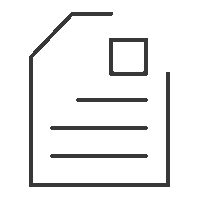
Advanced remediations driven by real-time anomaly detection
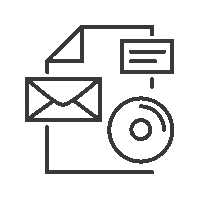
Centralized Rule Management for all data assets
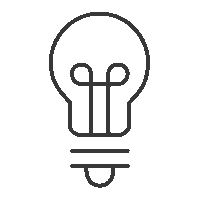
Comprehensive KPI Tracking Beyond the Basics
Take Our Interactive Product Demo
Build your DQ checks efficiently through automated, low-code, no-code, or full-code rules. Boost your coverage with automated rules.
Automated Technical & Business Rules
Qualytics leverages your historical data to build rich metadata and generate deep observability. From this context, the platform creates highly contextual technical and business rules that align with the natural shapes and patterns of your data. For a typical customer, approximately 95% of rules are automatically generated and continuously maintained by the platform.
Centralized Rule Management for all data assets
Unify technical and business rules in one platform. Qualytics centralizes data quality management for consistent enforcement, simplified governance, and seamless collaboration.
Advanced Rules and Custom Logic
Over 90% of data quality checks can be automated, but business logic often requires custom rules. That’s why Qualytics empowers both business users and data teams with low/no/full-code capabilities to easily define simple to highly complex rules. Not all checks are created equal. Advanced logic like aggregation comparisons, entity resolution, cross-datastore referential integrity, and data reconciliation require more sophistication—and Qualytics makes authoring these powerful checks both accessible and scalable.
Manual Rules
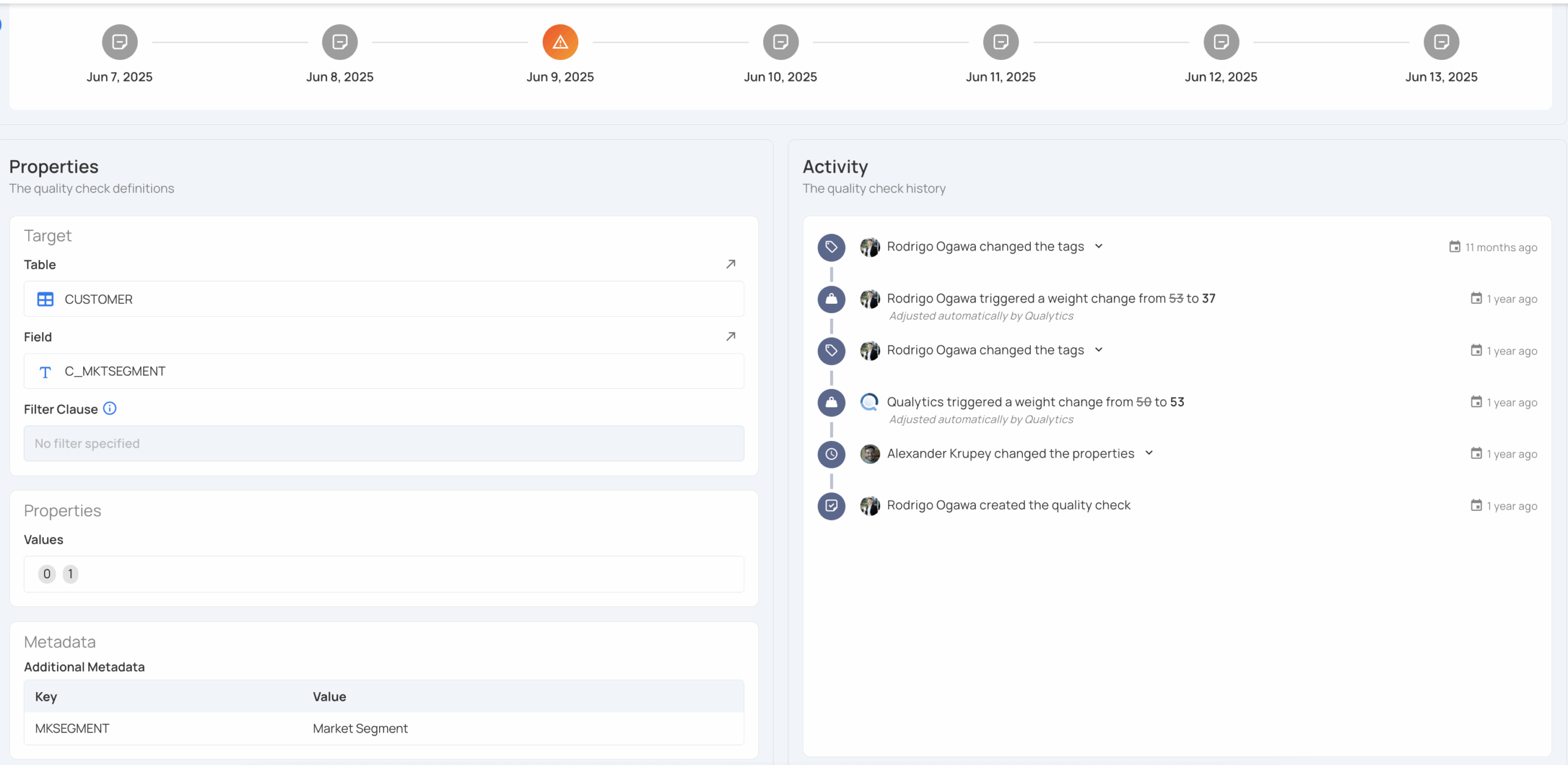
Reconciliation

Advanced remediations driven by real-time anomaly detection
Flexibly assert rules to detect anomalies at any stage of data ingress or egress. Drive downstream remediation using advanced Flows that orchestrate workflows based on specific data behaviors. Enable seamless access to anomalies and their contextual metadata through the Enrichment datastore.
Comprehensive KPI Tracking Beyond the Basics
Move past traditional SLA metrics like completeness and freshness by tracking all eight dimensions of data quality—accuracy, consistency, timeliness, validity, uniqueness, completeness, integrity, and conformity. With Qualytics, you can establish historical baselines, benchmark against expected norms, and generate customizable reports that surface trends and outliers over time. This allows teams to monitor quality across multiple datastores and data products, linking technical anomalies to business impact ensuring full transparency and accountability for data health at scale.
Solving Data Quality at Scale
Hear how leading data teams replaced traditional data quality methods with automated, scalable quality monitoring.
As a user of Qualytics, I am impressed by its robustness and high configurability.
Sabrina G.
Enterprise (> 1000 emp.)
Their approach is process oriented, and their tooling supports secure, cost effective approach that any size of company can get behind.
Verified User in Oil & Energy
Enterprise (> 1000 emp.)
Intuitive interface, powerful ML to create immediate data quality checks. Extremely responsive dev team.
Renee C.
Global Data Quality Lead at Revantage
Mid-Market(51-1000 emp.)
Frequently Asked Questions
What data stores does Qualytics support?
Qualytics supports any SQL data store and raw files on object storage. Popular data warehouses such as Snowflake, Databricks, Redshift; popular databases such as MySQL, PostgreSQL, Athena, Hive, along with CSV, XLSX, JSON and other files on AWS S3, Google Cloud Storage and Azure Data Lake Storage are a few examples of supported data stores. Users can also integrate Qualytics to their streaming data sources through our API.
How scalable is Qualytics?
Qualytics is architected with enterprise-grade scale in mind, and built on Apache Spark and deployed via Kubernetes. Through vertical and horizontal scalability, Qualytics meets enterprise expectations of high volume and high throughput requirements of data quality at scale.
Does Qualytics store my raw data?
Qualytics never stores your raw data. Raw data is pulled into memory for analysis and subsequently destroyed – anomalies identified are written downstream to an Enrichment datastore maintained by the customer. Highly-regulated industries may choose to deploy Qualytics within their own network where raw data never leaves their network and ecosystem.
What type of support is provided during the Onboarding process?
A dedicated Customer Success Manager, with mandatory weekly check-ins.
What types of data stacks does Qualytics support?
Qualytics supports both modern solutions and legacy systems:
- Modern Solutions: Qualytics seamlessly integrates with modern data platforms like Snowflake, Amazon S3, BigQuery, and more to ensure robust data quality management.
- Legacy Systems: We maintain high data quality standards across legacy systems including MySQL, Microsoft SQL Server, and other reliable relational database management systems.
For detailed integration instructions, please refer to the quick start guide.
What types of database technology can you connect in Qualytics?
Qualytics supports any Apache Spark-compatible datastore, including: – Relational databases (RDBMS) – Raw file formats (CSV, XLSX, JSON, Avro, Parquet)
Can I download my metadata and data quality checks?
Yes, Qualytics’s metadata export feature captures the mutable states of various data entities. You can export Quality Checks, Field Profiles, and Anomalies metadata from selected profiles into your designated enrichment datastore.
How is the Quality Score calculated?
Quality Scores measure data quality at the field, container, and datastore levels, recorded as a time series to track improvements. Scores range from 0-100, with higher scores indicating better quality.