Enabling Business Users
Every enterprise today is a data company. As data gets utilized for operational insights, competitive differentiators and beyond, domain expertise in subject matter is mission critical for success. Subject Matter Experts have the desire to own their data domains further while they work with centralized data governance and operations teams who desire to own systems and best practices.
However, the status quo of data quality – a fundamental part of data governance – is inherently broken. Business users struggle to establish fit with having to do constant back and forth with the data governance / data operations team to communicate needs and logic, and are not empowered to own the quality of their data better. This separation between data teams and business teams creates a chasm that we must cross if we are to scale data quality and data governance across our organizations.
According to Melody Chien, Senior Director Analyst at Gartner 1 .
“Data quality is directly linked to the quality of decision making. Good quality data provides better leads, better understanding of customers and better customer relationships. Data quality is a competitive advantage that D&A leaders need to improve upon continuously.”
This proves a critical need for change in how data governance is managed and utilized across the enterprise. In this article, we’ll explore the significant divide between the practices of data engineers and the operational insights of business users and will share how a more integrated & collaborative approach can significantly improve both data quality and decision making in your enterprise.
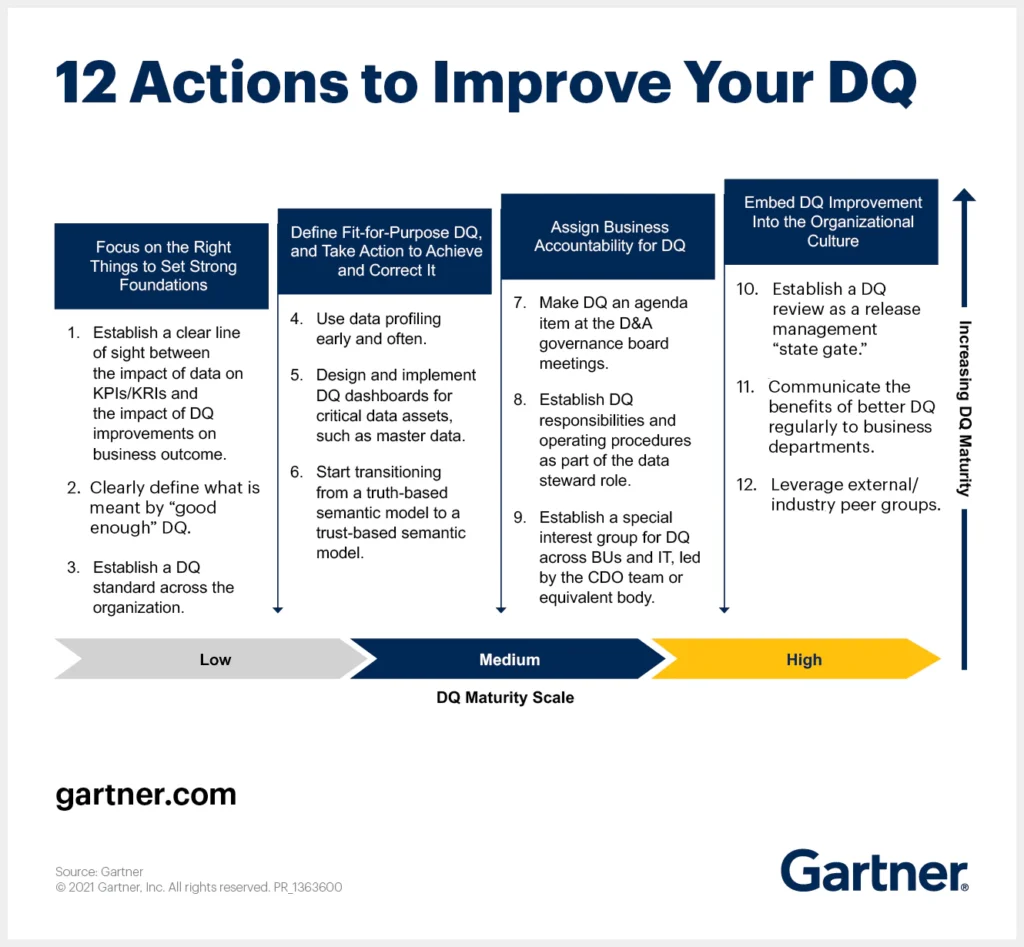
Understanding The Chasm In Data Quality
Let’s start by exploring the chasm between data engineers and business users. Business users are the Subject Matter Experts who have the deepest understanding of their data, from its expected patterns and behaviors to its overall structure. However, business users are often not expected to write code to implement business logic into a data quality control; they instead document business logic or an anomalous behavior as a request to a data team for development and production deployment, often after bad data has percolated into reports and dashboards.
On the opposite side of the chasm we have data teams – data engineers, data analysts, data scientists – who are responsible for implementing the business logic communicated by a business user into software. They often have limited context around the specifics of the business logic, and base decisions off requirements that have been documented. And as we all know, requirements are notorious for being changed often. This overall reactive process often leads to a vicious cycle of clarifications and revisions, not only slowing down processes but also hampering decision-making timelines that are crucial for business operations.
What if we empowered the business user to take ownership over data controls? What if business users could define their own rules and logic, review anomalies and their trends, and decide downstream workflow actions?
We call this Decentralized Data Quality, and it opens up endless opportunities for improved efficiency and results.
Crossing The Chasm With Decentralized Data Quality
Here are some of the key benefits of decentralizing your data quality by enabling the business user.
- Solidify a DQ Framework: Empowering business users with access to automated metadata to review statistical overview of data fields and ability to review automated data controls accelerates the first step in a DQ framework. This process establishes a baseline of data quality across the enterprise, provides gap analysis, defines KPIs for measurement and ultimately establishes DQ goals and standards.
- Establish Fit for Success: As a second step, business users should be allowed to author, manage, adjust, test, validate and productionize data controls based on their firsthand knowledge and logic directly into a DQ framework. Establishing fit for data’s expectations is the most crucial step in a DQ program – and empowering the business user to take ownership of this fit is crucial for DQ program success.
- Measure for Improvement: A decentralized DQ framework enables different business users to own DQ in their verticals or business units. Measuring improvement in DQ is directly correlated to KPIs defined, factors of DQ that matter to the business unit, and tracking such KPIs automatically over time.
- Find Innovative, Hidden Insights: Eliminating the chasm between business users with direct access to data and data controls empowers the business user to unlock previously unknown insights through reviewing metadata, statistical overviews, and ultimately anomalies caught. Bringing in the business user closer to data management ultimately fosters a culture of innovation to explore new ideas and insights that a centralized data team might overlook.
- Operationalize for Continuous Improvement: By enabling business users to directly engage with data quality processes, continuous improvement becomes part of everyday operations. This approach allows the SME and the data team to work together to quickly adapt and refine strategies, ensuring that data quality keeps pace with business needs. With this proactive model, improvements are constant rather than periodic, leading to more accurate and timely data that supports better business decisions and enhances operational efficiency.
Getting To Decentralized Data Management With Qualytics
At Qualytics, we’re built to superserve both business and data teams. Working with enterprises of various sizes, Qualytics recognizes the need for and delivers a comprehensive DQ framework, enabling teams to collaboratively manage their DQ end-to-end at scale as part of their Data Governance frameworks.
Qualytics creates a future where data quality management is seamlessly integrated into the core of business operations, bringing together business intelligence, data governance, and engineering teams in a unified effort – igniting a shift from reactive to proactive data quality management – ultimately building confidence in an enterprise’s data.
Qualytics empowers business users via automated profiling, automated data control inference, a low-/no-code User Interface with advanced rule authoring capabilities, and flexible automation of downstream remediation workflows. Qualytics is built to cross the chasm between data teams and business users.
If you’re interested in learning how this could impact the quality of your data and the efficiency of your processes, reach out to our team to get the no-obligation conversation started today.